Top 5 Aid Optimization Insights for Financial Aid Awareness Month
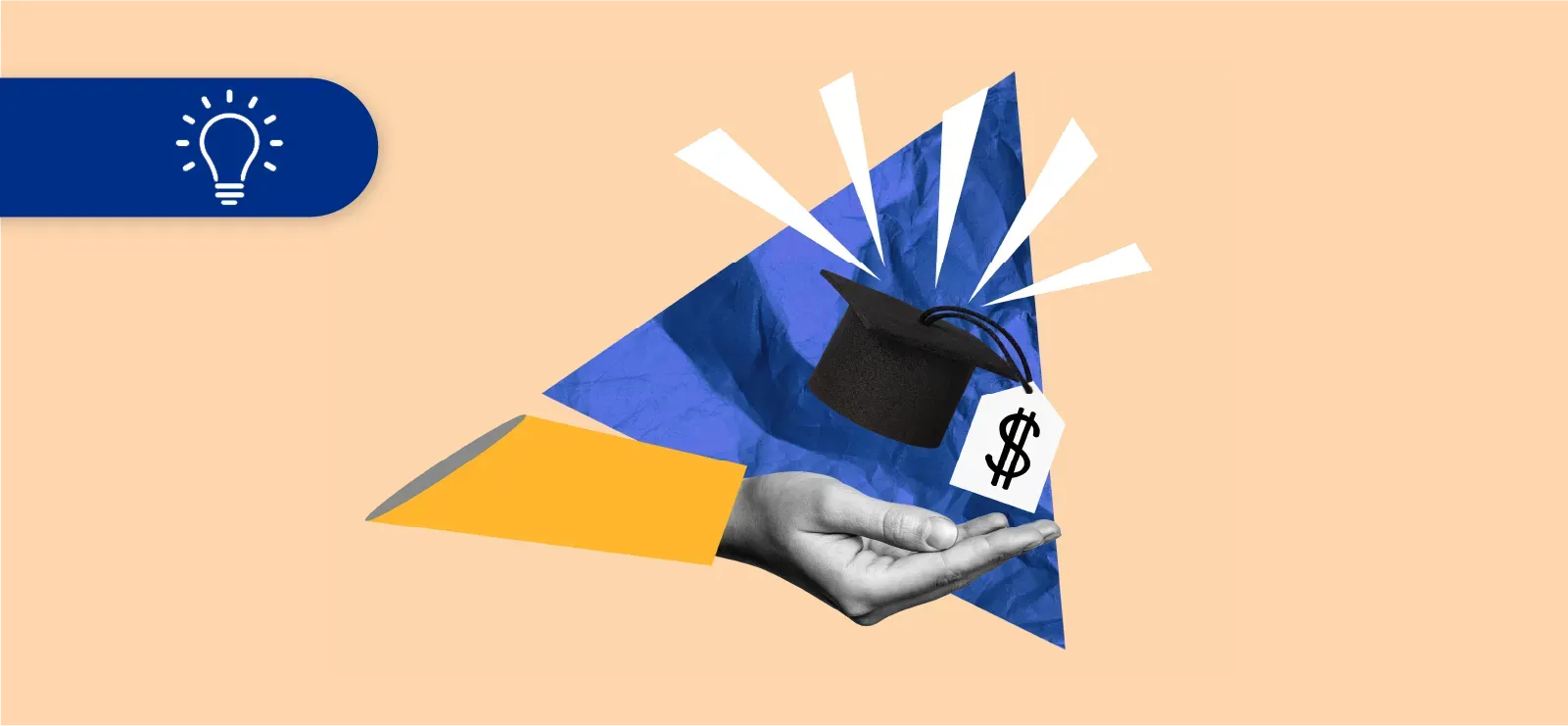
Optimized models are designed to be able to incorporate additional data sources beyond traditional leveraging models.
Financial aid leveraging is the established practice of a higher education institution using all financial aid resources at its disposal (federal, state, and institutional) to develop a strategy that helps a student to cross the line of affordability by maximizing the likelihood of enrollment for those students. Additionally, net tuition maximization is the by-product of this strategic approach, as the institution is also attempting to award the exact right amount to secure enrollment—without over-awarding. As a result, the institution maximizes its aid dollars at hand to provide as many opportunities as possible for their students.
Leveraging vs. Optimizing
One area that is emerging because of the growth of artificial intelligence tools now available to refine overall leveraging strategies is the concept of financial aid optimization. Financial aid optimization involves the continuous adjustments of aid dollars informed by real-time data to maximize student enrollments. Optimization moves an institution’s leveraging strategy forward in five key ways.
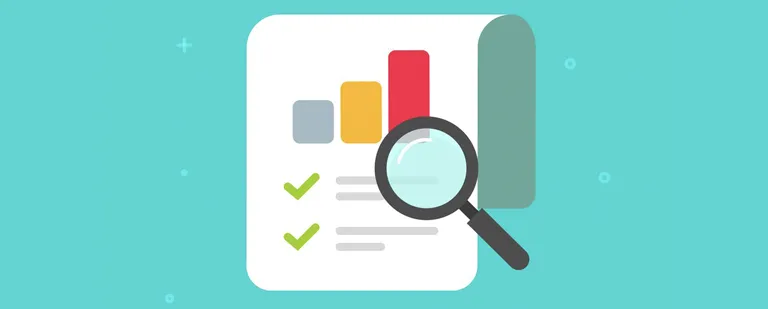
Source: iStock
1. Optimization is not a set-it and forget-it process.
Leveraging strategies tend to involve significant analysis and may take several years to fully implement. However, due to the desire to test the model in a typical leveraging approach, changes are usually made once a year after the class has started and the analysis of what did or did not work has been completed. One key differentiator of aid optimization is that it occurs in real time, allowing the enrollment professional to adjust a base leveraging strategy regularly depending on the time of the enrollment cycle, student characteristics, or changes that are occurring.
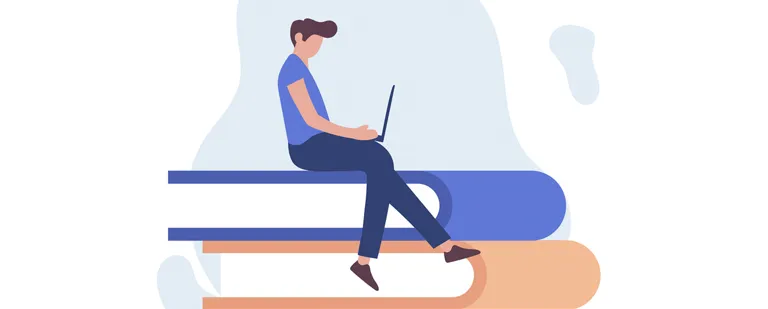
Source: iStock
2. Optimization exists at the individual and cohort levels.
Leveraging models, especially within larger institutions, require the need to assess students and student groups (cohorts) together to effectively balance the workload and the systems programming requirements necessary to efficiently award aid. However, an optimized model takes the base leveraging approach a step further by drilling down to an individual student in real time. As a result, aid appeals, competitive offers, or one-time foundation funds that become available unexpectedly for a particular department or college, can be effectively used in an optimized model to maximize enrollments.
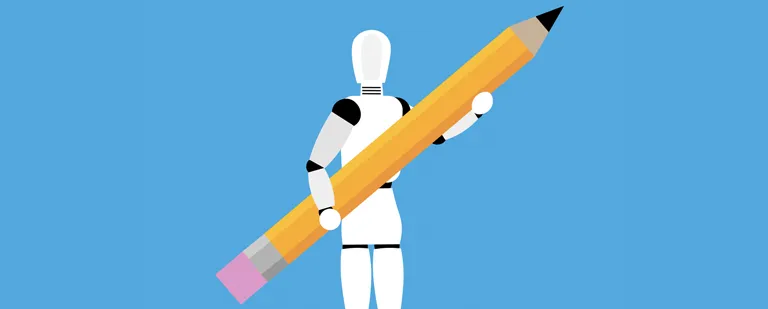
Source: iStock
3. AI optimization is not a chatbot.
In much the same way that “Kleenex” has become a genericized term for all tissues, “artificial intelligence” has become a catchphrase for anything that resembles some form of automation, especially in the enrollment space. And while the use of AI-informed chatbots may play a role in some strategies, such as ideation, it is not a key component of aid leveraging. Annual leveraging models tend to rely on deep-level analytics—with frozen data sets looking back in time—and then use that data to predict the future state. On the other hand, optimized machine learning, that is truly prescriptive, identifies how to award the right amount of aid at the right time to the right student to maximize enrollment. Through a fully integrated machine learning model, optimized aid models can be more fully aligned to campus-wide goals. For example, such a model can prescribe the next best steps in financial aid awarding and the outreach necessary to align campus and enrollment goals.
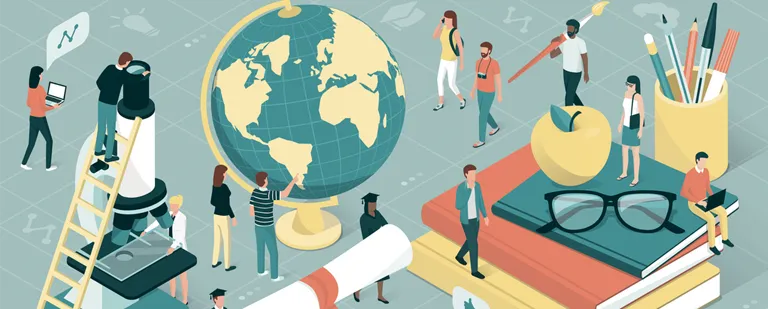
Source: iStock
4. Optimizing goes further than just enrolling a new student.
Optimized models are designed to be able to incorporate additional data sources beyond traditional leveraging models. Does your model for awarding aid account for how many students swiped into the library last year? With an effective optimized model, you can use that type of data to inform your understanding of the characteristics that are shown to enhance retention and persistence. For instance, you can identify which factors increase the likelihood of retention on your campus and use that information to derive a likelihood-to-retain analysis to prioritize aid awarding. Even better, you can cite that data to inform campus leaders that there will need to be more resources available next year to support student persistence in light of the way the new class is shaping up and your corresponding campus needs.
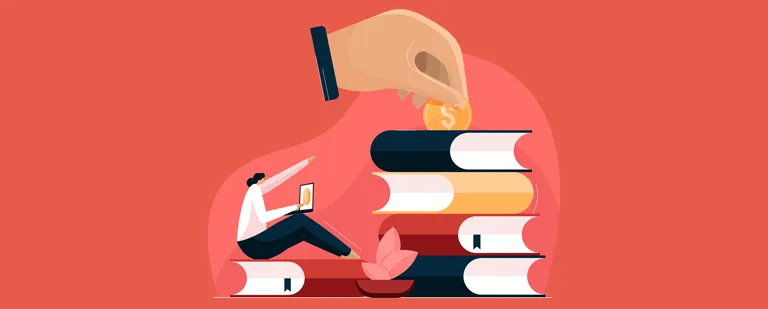
Source: iStock
5. Optimization delivers just when you need it.
An optimized aid strategy is marked by continual machine learning that only gets better over time. It improves not only year over year, but especially during yield season when a few key decisions or adjustments can make all the difference between meeting (and surpassing) enrollment goals or falling short. The optimized model can consider, in real time, a sizable number of inputs on each student. Through those multi-level, multi-interlaced inputs, it can then help determine the best financial aid prescription to maximize the likelihood to yield that student. This key information makes it possible to shape a class in ways that were previously unavailable, even with a team of intuitional research staff running pivot tables.
Financial aid leveraging models are a significant and necessary component of any strategic enrollment plan. However, they are limited in their impact when they are not optimized. An effective AI machine-learning predictive and prescriptive approach can suggest adjustments in real time based on the goals that have been set, applicant behaviors, and the myriad of changes that can occur in any enrollment year.
Perhaps you’ve looked at end-of-year data and said, “I wish I had known that. I could have done something about it.” An optimized aid strategy can give you not only crucial information, but also confidence that your affordability concerns, even at the individual student level, have been addressed as well as possible. That, in turn, allows you to focus on the other issues necessary to meet and exceed your enrollment goals.